Learning Dexterous Manipulation
Learning dexterous hand manipulation skills through imitation or reinforcement learning.
We trained reinforcement learning (RL) for dexterous robotic hands motions and brought it to the real world, For this, we introduced the biomimetic tendon-driven Faive Hand and its system architecture, which uses tendon-driven rolling contact joints to achieve a 3D printable, robust high-DoF hand design. And we model each element of the hand and integrate it into a GPU simulation environment to train a policy with RL, and achieve zero-shot transfer of a dexterous in-hand sphere rotation skill to the physical robot hand.
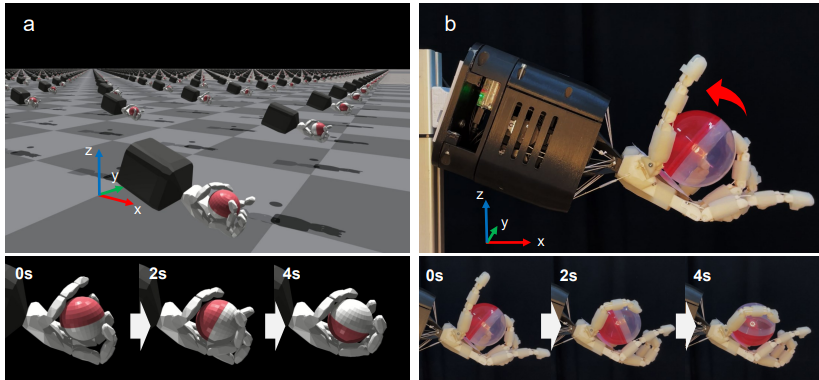
We apply imitation learning to dexterous robotic hands. To bridge the gap between the high complexity of the manipulation task and the lack of the demonstration dataset designated to the robotic hand, we propose a method to leverage multiple largescale task-agnostic datasets to obtain latent representations that effectively encode motion subtrajectories. Our results demonstrate that employing latent representations yields enhanced performance compared to conventional behavior cloning methods, particularly regarding resilience to errors and noise in perception and proprioception. Furthermore, the proposed approach solely relies on human demonstrations, eliminating the need for teleoperation and, therefore, accelerating the data acquisition process.
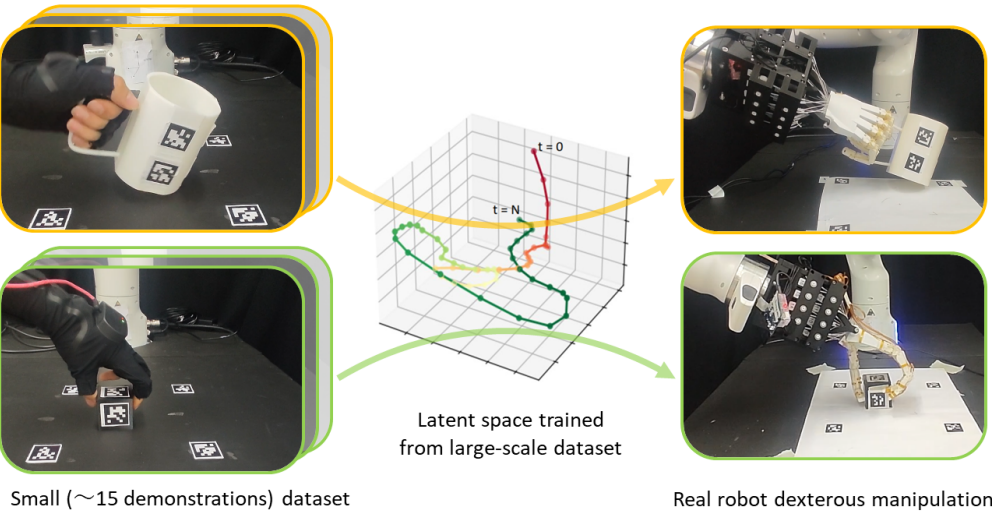