Modeling and Control
Building on previous work on soft robotic arms, we develop computationally efficient physics models that capture only the relevant degrees of freedom of a soft robot. Our short-term goal is to pursue dual research tracks to achieve a universal modeling framework. For symmetric robots, we postulate that minimal parameter methods are sufficient to capture the dynamics and impedance of the deformable structures while being computationally efficient. For robots of irregular shape, we develop large scale finite element methods and make those models tractable by expanding on techniques such as model order reduction with state observers and deep learning methods. The creation of a modeling framework will be accelerated through active collaborations with faculty working on numerical simulations of physical systems, and researchers working on the modeling software external pageSOFAcall_made and the dynamical systems toolbox external pageDrakecall_made.
Control Techniques Enabling Complex Behaviours
We conceive model-based controllers and reinforcement learning techniques that can perform difficult tasks requiring interaction with a robot’s surroundings. Our research was one of the first explorations into dynamic closed-loop control of soft continuum robots, with a short-term goal to generalize the current modeling approach to encapsulate non-linear deformations of hyperelastic materials in real-time. Collaborating with colleagues across faculty boundaries on developing new control strategies for soft robotic systems. The long-term goal is to use the model-based controllers as basic building blocks in a reinforcement learning framework for the development of advanced shapeshifting and autonomous manipulation capabilities for dexterous tasks.
DeSKO: Stability-Assured Robust Control with a Deep Stochastic Koopman Operator
The Koopman operator theory linearly describes nonlinear dynamical systems in a high-dimensional functional space. This facilitates the application of linear control methods to highly nonlinear systems. However, the Koopman operator does not account for any uncertainty in dynamical systems, leading to fragile control performance in real-world applications.
We therefore propose a deep stochastic Koopman operator (DeSKO) model in a robust learning control framework to guarantee stability. The DeSKO model can learn the uncertainty contained in the dynamical system and infer a distribution of observables. We use the inferred distribution to design a robust and stabilizing closed-loop controller for the dynamical system. Modeling and control experiments on several advanced control benchmarks show that our presented framework is more robust and scalable than the state-of-the-art deep Koopman operators and reinforcement learning methods. Tested benchmarks include a soft robotic arm, a legged robot, and a biological gene regulatory network. We further demonstrate that this robust control method resists previously unseen uncertainties, such as external disturbances, with a magnitude of up to five times the maximum control input. Our approach opens up new possibilities in learning control for high-dimensional nonlinear systems while robustly managing internal or external uncertainty.
M. Han, J. Euler-Rolle, R. K. Katzschmann. "external pageDeSKO: Stability-Assured Robust Control with a Deep Stochastic Koopman Operatorcall_made". International Conference on Learning Representations(ICLR), April, 2022.
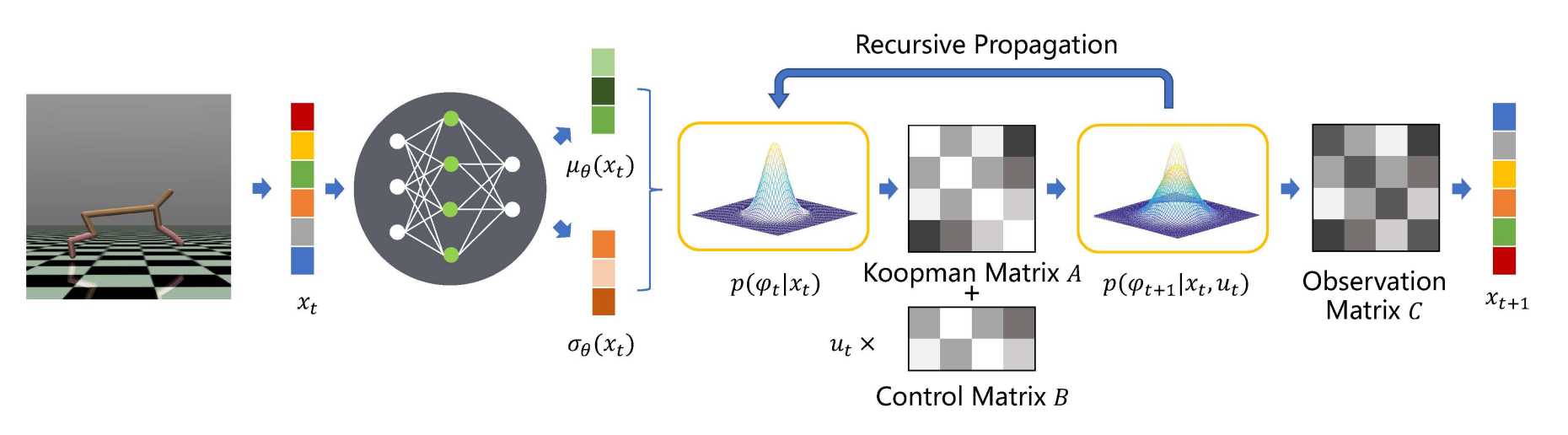
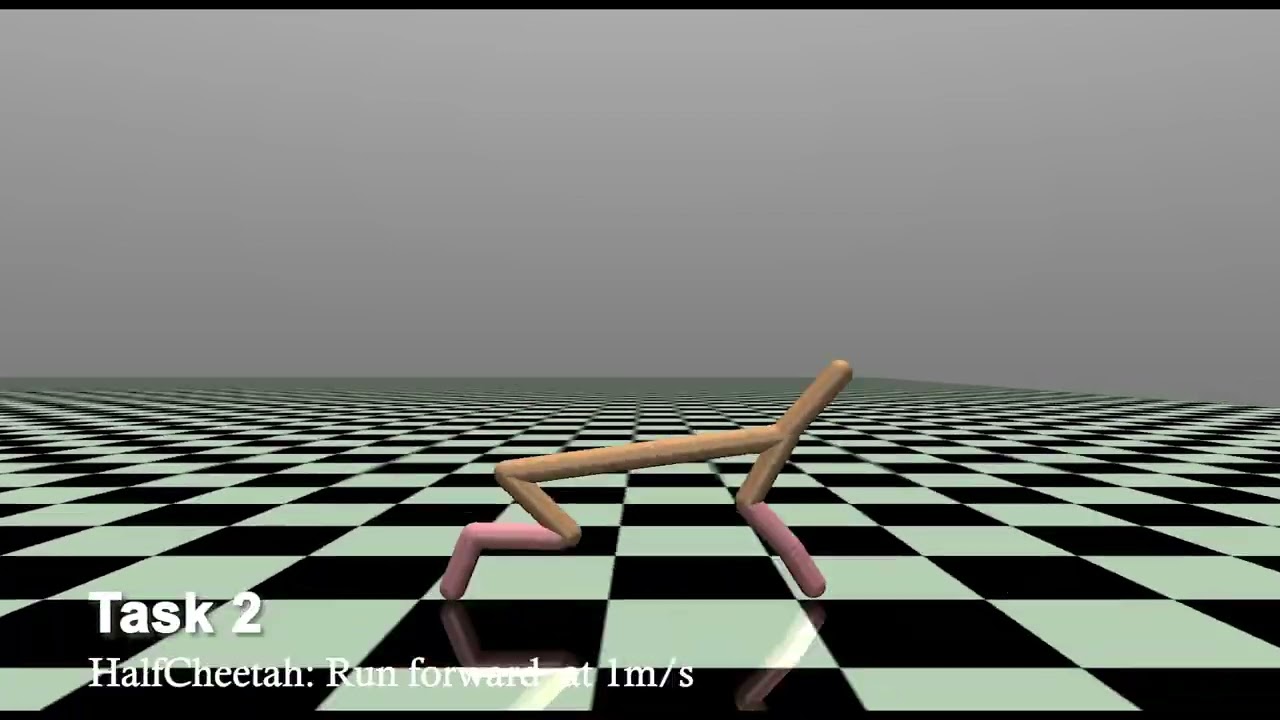
Learning Material Parameters and Hydrodynamics of Soft Robotic Fish via Differentiable Simulation
The high dimensionality of soft mechanisms and the complex physics of fluid-structure interactions render the sim2real gap for soft robots particularly challenging. Our framework allows high fidelity prediction of dynamic behavior for composite bi-morph bending structures in real hardware to accuracy near measurement uncertainty. We address this gap with our differentiable simulation tool by learning the material
parameters and hydrodynamics of our robots. We demonstrate an experimentally-verified, fast optimization pipeline for learning the material parameters and hydrodynamics from quasi-static and dynamic data via differentiable simulation. Our method identifies physically plausible Young’s moduli for various soft silicone elastomers and stiff acetal copolymers used in creation of our three different fish robot designs.
For these robots we provide a differentiable and more robust estimate of the thrust force than analytical models and we successfully predict deformation to millimeter accuracy in dynamic experiments under various actuation signals. Although we focus on a specific application for underwater soft robots, our framework is applicable to any pneumatically actuated soft mechanism. This work presents a prototypical hardware and simulation problem solved using our framework that can be extended straightforwardly to higher dimensional parameter
inference, learning control policies, and computational design enabled by its differentiability.
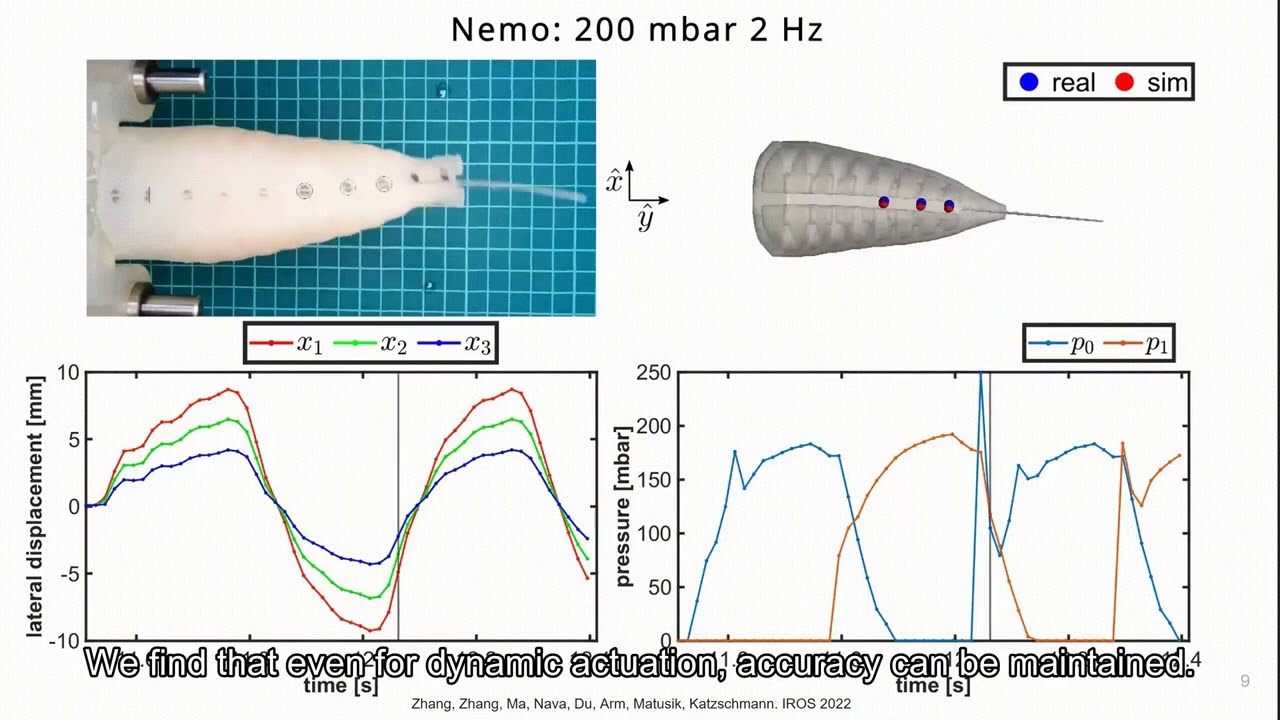