Robotic Control
Control techniques enable complex behaviours on continuum robots.
DeSKO: Stability-Assured Robust Control with a Deep Stochastic Koopman Operator
The Koopman operator theory linearly describes nonlinear dynamical systems in a high-dimensional functional space. This facilitates the application of linear control methods to highly nonlinear systems. However, the Koopman operator does not account for any uncertainty in dynamical systems, leading to fragile control performance in real-world applications.
We therefore propose a deep stochastic Koopman operator (DeSKO) model in a robust learning control framework to guarantee stability. The DeSKO model can learn the uncertainty contained in the dynamical system and infer a distribution of observables. We use the inferred distribution to design a robust and stabilizing closed-loop controller for the dynamical system. Modeling and control experiments on several advanced control benchmarks show that our presented framework is more robust and scalable than the state-of-the-art deep Koopman operators and reinforcement learning methods. Tested benchmarks include a soft robotic arm, a legged robot, and a biological gene regulatory network. We further demonstrate that this robust control method resists previously unseen uncertainties, such as external disturbances, with a magnitude of up to five times the maximum control input. Our approach opens up new possibilities in learning control for high-dimensional nonlinear systems while robustly managing internal or external uncertainty.
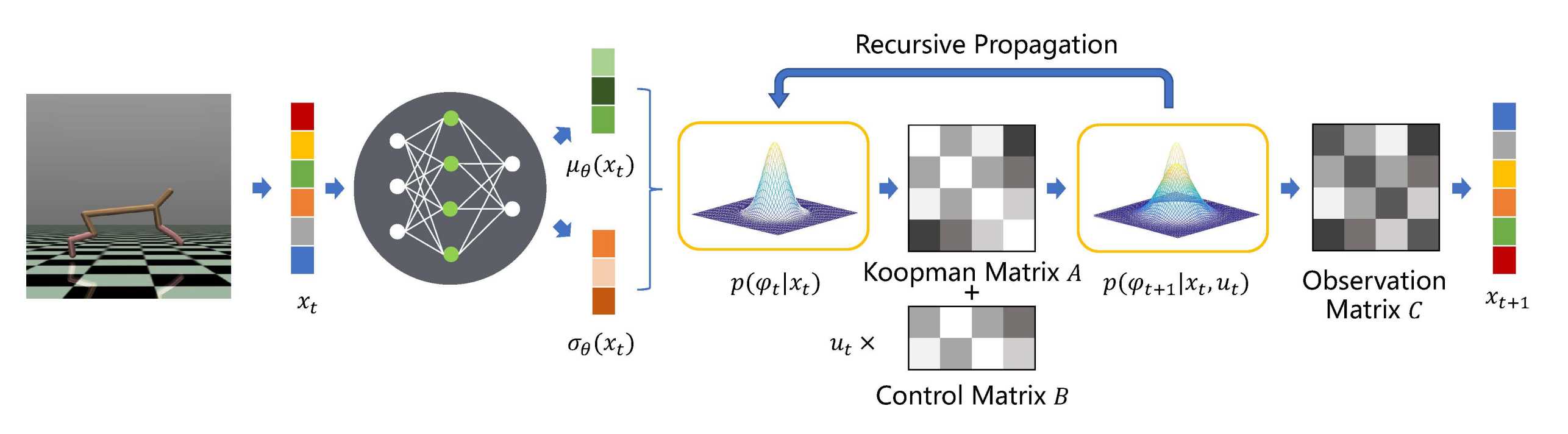